Some of you have contacted me about the recent CTV and Globe news tories about an extra-ordinarily poor scientific paper and its erroneous conclusions that was drafted by Dr. David Fisman, one of his colleague and a graduate student. We discussed the many deficiencies of this publication at the Canadian Covid Care Alliance Scientific and Medical Advisory Committee meeting this evening, and we will be preparing a more in-depth critique of this article in the coming days. One of our Committee members, Dr. Byram Bridle has already produced an opinion piece, which I am sharing with you below.
Dr. Byram Bridle’s interview with Rebel News. The facts printed below, so you can check them yourself.
Go directly to Dr Brindle’s article, at the bottom of this page by clicking here
Dr Steven Pelech’s etal paper click here
Additional comments by Ted Kuntz click here
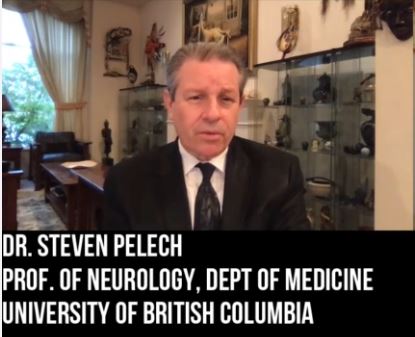
In addition, members of our Committee wrote a scientific publication that was accepted today for publication in a peer-reviewed journal. McLeod, D., Martins, I., Pelech, S., Beck, C., Shaw. C.A. (2022) Dispelling the myth of a pandemic of the unvaccinated. Int. J. Vaccine Theory Practice Res. (in press). I have attached a copy of the pre-print of this article. Even though the manuscript was written over a month ago, it further explains why the Fisman publications is so wrong in its pronouncement that COVID-19 is being driven by the vaccinated.
🙂 Best wishes from Steven Pelech.
Dispelling the Myth of a Pandemic of the Unvaccinated
Deanna McLeod, HBSc, Kaleidoscope Strategic,
deanna@kstrategic.com
Ilidio Martins, PhD, Kaleidoscope Strategic,
ilidio@kstrategic.com
Steven Pelech, PhD, Kinexus Bioinformatics Corporation;
Department of Medicine, University of British Columbia,
Vancouver, BC, Canada, spelech@mail.ubc.ca
Ceilidh Beck, BHK
Christopher A. Shaw, PhD, Department of Ophthalmology and Visual
Sciences, Program in Neuroscience; Program in Experimental
Medicine, University of British Columbia, Vancouver, BC, Canada,
shawc@ubc.ca
Abstract
We have reanalyzed the Ontario Science Table data on hospitalizations for COVID-19 in COVID-19 vaccinated versus unvaccinated patients admitted to hospital during various waves of the pandemic. In spite of the Ontario Science Table and the mass media claims that the unvaccinated were the dominant population being hospitalized, a more rigorous evaluation of the existing data shows that this narrative is not correct for the latter waves of COVID-19. We identify a series of methodological issues that may have lead the Ontario Science Table to reach their conclusions and explain why such issues may have led to policies that have been largely ineffective.
Key words:
Ontario Science Table, hospitalizations due to COVID-19, pandemic of the unvaccinated, methodological errors
Introduction
According to various reports in the mainstream media, Ontario health data show that those unvaccinated against COVID-19 are up to 60-times more likely to end up in intensive care units (ICUs) with COVID-19 than the vaccinated (Young, 2021; Dunham, 2021; Morgan, 2021). With alarming headlines, the media promoted a narrative that the unvaccinated are driving the pandemic and increasing the burden on ICUs in Ontario at the expense of other people. These headlines typically depict the unvaccinated as careless vectors of disease and thus potentially dangerous and hence responsible for society’s current COVID-19 plight. These headlines and the ensuing health policy, fails to recognize that at this stage of the COVID-19 pandemic, at least 22% and like more than 50% of the Canadian public appear to have been exposed to SARS-CoV-2, recovered, and developed natural immunity(Canadian Blood Service, 2022; Parsons, 2022; Michael Kuzmickas, personal communication; S.P., personal communication).
The vaccines in question are those currently in use in Canada where two of six use mRNA platforms, and two of the others use adenovirus-based delivery of DNA, from which mRNA is generated. Each of these four vaccines ultimately produce the spike protein of SARS-CoV-2, which is presented on the surfaces of cells near the injection site. Emerging bio-distribution data clearly show that both the spike protein produced and even some of the mRNA construct may migrate to sites far from the injection site (Comirnaty. European Medicine Agency Assessment, February 19, 2021; https://www.ema.europa.eu/en/documents/assessmentreport/comirnaty-epar-public-assessment-report_en.pdf).
Because such assertions about the COVID-19 unvaccinated have far-reaching consequences, for both medical treatments as well as civil rights, there is a growing need for a critical evaluation of the accuracy of the various claims, particularly given that they can lead to increasing stigmatization from both the public and various levels of government against those who
have chosen to not undergo vaccination against COVID-19. This analysis of the Public Health Ontario and Government of Ontario, Ministry of Health COVID-19 databases will focus on four areas of concern: the questionable basis for mass vaccination, the methodology of the studies used to support current vaccination policies, the lack of effectiveness of those same policies, and how these have failed to actually to consider vaccine safety with the hopes of supporting informed decision making at both individual and health policy levels.
Methods
Total and daily COVID-19 cases, total hospitalizations and deaths data were obtained from the “Full COVID-19 Summary Data for Ontario” datasheet available at the Public Health Ontario (PHO) COVID-19 Data Tool webpage (Public Health Ontario, 2022b) on January 5, 2022. In this dataset, hospitalization numbers included all cases reported as ever being hospitalized during their infection. Daily hospitalizations were calculated from the difference between total hospitalizations for the index date and the previous day. Similarly, the number of daily deaths were calculated from the difference between total deaths reported for the index date and the previous day. The number of daily COVID-19 cases, case rates (per 100,000, 7-day average), individuals in intensive care units (ICU) and in hospital (excluding ICU) due to COVID-19 by vaccination status, and individuals with at least one, two (considered fully vaccinated at the time) or three (boosters) vaccine doses were obtained from the “COVID-19 Vaccine Data in Ontario” datasheets as cited above.
The number of individuals in ICU’s and in hospitals excluding ICU’s were combined in order to obtain the total number of individuals in hospital due to COVID-19 by vaccination status. Daily mean temperature at Toronto International Airport was obtained from the Government of Canada, Historical Weather and Climate Data(webpage for the TORONTO INTL A, ONTARIO station; Government of Canada, 2022) on January 5, 2022).
Data from all sources were combined, organized, processed, and plotted in MS-Excel. While index dates for the Government of Ontario Data Catalogue were reporting dates with numbers indexed to the day in which numbers were released, PHO data were indexed to the day prior (of data collection). This caused a one-day shift between datasets that became apparent when trying to use them together. To harmonize the datasets, data collection dates were used as index dates for both datasets.
Results
Some potentially invalid assumptions driving vaccine policy
Antibody Efficacy
Most tests of antibody efficacy are based on assessing the extent to which vaccinal antibodies interfere with the binding of the SARS-CoV-2 spike protein to the host cell ACE2 receptor. In the rapidly emerging COVID-19 variants, mutations within the receptor binding domain may enhance the binding to ACE2, but reduce recognition by specific antibodies that target this region. However, immune responses are directed against many different regions of the spike protein, and binding of antibodies to these regions can also confer significant immune protection. This may include facilitating recognition of the spike protein and virus by immune cells that feature Fc receptors for the constant region of immunoglobulins as well as by the complement system. In any event, it is misleading to insist, as often the media or the OST do, that mass vaccination with COVID-19 vaccines will be effective over the long term or that they will be a means of achieving herd immunity. This becomes even more problematic when failure of the vaccines to block transmission is blamed on the unvaccinated.
Mass Vaccination
The practice of mass vaccination is based on the notion of “herd immunity”, that is when a high percentage of the population has either contracted and recovered from any particular disease, thus gaining natural immunity, or has gained sterilizing immunity through specific vaccinations. At herd immunity, modeling data indicates that the disease cannot spread further and should not be transmittable to those who have neither form of immunity (e.g., unexposed or immunocompromised persons) (Shaw, 2021). The thresholds for attainment of herd immunity vary depending on many factors including characteristics of the virus, population, and vaccines. Early modelling of COVID-19 vaccine impact showed that herd immunity should be achievable when only 55% of the population has been vaccinated under
reasonably favorable conditions (Hogan & Winskil et al., 2020; Phillips, 2021). It should be noted that although the
traditional definition of herd immunity included both natural and vaccine-derived immunity, at the outset of the COVID-19 pandemic the World Health Organization changed the definition of herd immunity to something that is achieved almost entirely through vaccination (WHO, 2020).
It is also worth noting that historically, vaccination alone has not been routinely successful in providing long lasting herd immunity for some diseases, due to secondary vaccine failure (Mathias & Meekison et al., 1989; Edmonson & Addiss et al., 1990; Zhang & Chen et al., 2018; Connell & Connell et al., 2020. The latter may occur as immune responses in terms of levels of antibody levels, T cells, and even memory cells decline over time (Heffernan & Keeling, 2008; Duintjer Tebbens & Pallansch et al., 2013; Vygen & Fischer et al., 2013; Koopman & Henry et al., 2017; Burdin & Handy et al. 2017; Lewnard & Grad, 2018). In addition, the infectious pathogen may undergo extensive mutation that may compromise immune recognition.
The long-lasting success of any vaccination campaign in halting disease is almost exclusively dependent on the use of vaccines that confer sterilizing immunity and do not suffer rapid secondary vaccine failure (Hellerstein, 2020; Pollard & Bijker, 2021; Yewdell, 2021; Focosi & Maggi et al., 2022). In contrast, the two doses of novel mRNA COVID-19 vaccines by Pfizer or Moderna or the viral vector vaccine of AstraZeneca, in contrast to those derived from conventional vaccine platforms, seem to exhibit a relatively rapid decline in efficacy, and as such can be characterized as “leaky”, with outbreaks occurring over short time periods even in those with boosted vaccinations (Scott & Richterman et al., 2021). In addition, it is increasingly clear that individuals receiving COVID-19 vaccines can become reinfected or experience breakthroughs, as well as transmit the virus to others (Read & Mackinnon, 2008; Keeling & Tildesley et al., 2013; Read & Baigent et al., 2015; Brown & Vostok et al., 2021). Moreover, any efficacy initially promoted for these vaccines was based on PCR-confirmed symptomatic infection against the original Wuhan strain, and we currently lack proper assessment of transmission-blocking efficacy of the vaccine against emerging COVID-19 variants (Collie & Champion et al., 2021; Dejnirattisai et al.; Shaw et al., 2021; Keegan & Truelove et al., 2021; Lopez Bernal & Andrews et al., 2021; Reis & Barda et al., 2021; Singanayagam & Hakki et al. 2021; Thiruvengadam & Awasthi et al., 2021; Torgovnick, 2021).
Concerns Regarding Study Methodology of Supporting Evidence
The strength of a medical recommendation should rest on the level of evidence on which it is based. The highest level of evidence comes from a double-blinded, randomized Phase III trial showing benefits against a standard of care in a specific population (Misra, 2012). In contrast, retrospective analyses are unable to control for the influence of confounding variables and thus are more effectively used to generate hypotheses that can later be validated in prospective controlled trials. Such analyses are not sufficiently reliable to support mandatory treatment recommendations (Talari & Goyal, 2020). For example, retrospective analyses must be appropriately interpreted by considering the completeness of the data set, the terminology used, and the monitoring protocols, as well as the methods employed and verifiable assumptions.
Data Completeness
Population based COVID-19 registries are designed to collect data on COVID status, but not data on hospitalization events (Government of Ontario, 2022; Government of Ontario, 2021). Presently we know that the Canadian COVID-19 database preferentially captures data on symptomatic individuals and inevitably underrepresents those who refuse or are not required to undergo testing as well as those who are asymptomatic (Public Health Ontario, 2022b). For this reason, any such results should be extrapolated with caution when considering mildly symptomatic or asymptomatic individuals.
Conducting an analysis on COVID-19 hospitalization outcomes would require merging the COVID-19 database with one that is able to capture hospital level events, and as such the completeness of hospital data captured would need to be taken into consideration to ensure the reliability of the outcomes. Likewise, data would need to be interpreted in light of differences in monitoring or sampling across vaccination groups. Comparing outcomes across vaccination groups, however, assumes these groups are homogeneous which is unlikely. For example, it would not be unexpected for the groups to differ in terms of natural immunity status, virus exposure, and risk of severe disease. In Toronto, from January 1st to July 31st 2020, there were just under 1,000 hospitalized COVID-19 cases in the 70+ age group and just over 600 hospitalizations in the 50-69 age group (City of Toronto, 2022). Aside from age, underlying medical conditions such as “obesity, diabetes with complication and anxiety disorders were the strongest risk factors for severe COVID-19 illness”(Kompaniyets & Pennington et al., 2021). Since details about other potentially relevant variables were not provided by Public Health Ontario, these omissions reinforce the view that caution should be used when ascribing differences in observed outcomes to vaccination status alone.
Terminology
Another factor to consider is the definitions used to describe vaccination status. Public Health Ontario defines vaccination status based on immunity achieved 14 days after any second dose (Ontario Ministry of Health, 2021), contradicting at least one company using an mRNA platform, Pfizer, that in the Phase III trial asserted that immunity can be established after 7 days (Thomas & Moreira et al., 2021). From such discrepancies, it seems likely that fully vaccinated individuals will be considered partially vaccinated up to 14 days after their vaccine, and individuals receiving their first dose will typically be categorized as unvaccinated up to 14 and even 21 days later. These overlapping definitions confound interpretation of outcomes as many vaccinated individuals will be classified as unvaccinated, thus inflating the numbers of the latter and making it difficult to distinguish differences in disease status and health outcomes (e.g., infection, hospitalization, deaths) among vaccinated and unvaccinated. It would have been better if the unvaccinated category had excluded those who have been vaccinated.
Monitoring
The main test used to date in Ontario and elsewhere in Canada is the PCR (Polymer Chain Reaction) assay, a test which detects genetic fragments of the SARS-CoV-2 virus in tested individuals. Of note, with a cycle threshold (Ct) (the number of times the sample is amplified) typically used in Covid-19 testing (35 and above), the possibility of false positivity and thus actual infectivity becomes highly problematic (Mina & Peto et al., 2021). This limitation is now acknowledged by the Centers for Disease Control and Prevention (CDC) (Centers for Disease Control and Prevention, 2021). For example, a Ct cut-off for positivity over 35 (Public Health Ontario officially uses a Ct of 35) (Public Health Ontario, 2021a) may result in up to an 85% false positive rate (Public Health Ontario, 2020). If these Ct levels are then used to describe “cases”, and asymptomatic low probability persons comprise most of tests conducted, the resulting data will inevitably generate inflated case numbers in that population. Moreover, someone with COVID-19 may only be infectious between 4 and 8 days, but may test “positive” between 22 and 33 days, introducing the possibility that a single infection may be recorded as more than one case (Mina & Peto et al., 2021).
Given our current vaccine mandate environment, unvaccinated individuals often have to undergo systematic asymptomatic testing by their employers (CBC News, 2021; Government of Ontario Ministry of Health, 2021; Herhalt, 2021; Pringle, 2021). This increase in sampling is likely to result in higher case counts in this population where Ct levels are likely to generate greater false positives. In addition, as cited above, data collection systems may not be able to eliminate multiple counts of the same infection (Goodfield, 2021; Public Health Ontario, 2021b). Further, it remains unclear whether there are differences in testing requirements between hospitalized individuals based on vaccination status. Added to these constraints, it is well established that SARS CoV-2 is transmitted more readily in communal living environments such as hospitals (Morawska & Tang et al., 2020). Thus, when interpreting the data, it is important to distinguish between individuals who were in the hospital for some non-COVID-19 related reason and then test positive for SARS CoV-2, compared to those who are in the hospital due to a COVID-19 infection. Furthermore, as it is often unclear what Ct was used and whether there were differences in monitoring based on vaccination status, the Ontario COVID-19 data showing differences between the vaccinated and unvaccinated is likely to be unreliable.
Study Design and Analysis
Retrospective reviews of the COVID-19 database are helpful for identifying potential associations between various events, but as they cannot control for differences in baseline factors, monitoring, or exposure, they are unreliable (Mann, 2003; Ranstam, 2019). Most importantly, the ability of retrospective analyses to infer causality is limited, and therefore should not be used to support major health policy decisions.
COVID-19 Risk Factors
There are some well-established factors that increase the risk of severe COVID-19 outcomes. These include older age, obesity, diabetes co-morbidities (Kompaniyets & Pennington et al., 2021; Kompaniyets & Goodman et al., 2021). Factors that lower the risk of severe disease includes naturally acquired prior immunity (Cohen & Linderman et al., 2021) and younger age (Woolf & Champman et al., 2021), as well as access to pharmacological interventions. As analyses are not always randomized and do not control for baseline factors, differences in such factors in vaccinated and unvaccinated populations could be partially, or fully, driving the claimed effects of higher unvaccinated cases being causal to hospitalizations and deaths. For instance, frail elderly individuals are often not vaccinated due to a risk of vaccine injury. However, it would not be unreasonable, given the higher levels of co-morbidities associated with this group, to see a greater proportion of these individual in hospitals or ICU units, which may be misinterpreted as the unvaccinated being at greater risk of severe COVID-19 outcomes.
Concerns Regarding Effectiveness of Current Vaccination Policy
There has been some discrepancy concerning how many COVID-19 “waves” have occurred since the onset of the pandemic in early 2020. For the purposes of this article, while there has been five distinct waves starting with the initial wave in the spring of 2020, we have focused on the latter four waves. The second wave transpired from Nov 1, 2020 to January 4, 2022 (Figure 1. The second and third waves peaked above 4,000 cases per day in Ontario and occurred over the colder months beginning in November 2020, declining with warmer summer weather. Unlike the second and third waves, the fourth wave began at the height of the warmer summer weather in August, with an initial rise up to 1,000 cases per day in early September 2021, before dramatically shooting up in a fifth wave to an unprecedented 18,000 cases per day by early January 2022, the latter with a more than 4-fold increase over prior peaks. Whether the Omicron B.2 variant is emerging a sixth wave is presently unclear and not essential to the analysis below.
As noted above, herd immunity should be achieved with a highly effective vaccine once some 50% of the population is immune from prior infection or vaccination. In this regard, it should be noted that the third wave was clearly receding before the 50% vaccination threshold had been reached for the first shot in early May 2021. Further, the shape of the decline of the curve did not change after this threshold was achieved. Such data indicates that natural immunity rather than vaccine-induced immunity was the main driving factor in the decline of the third wave.
By early August 2021 at least 65% of the population had been fully vaccinated by the definition of the time, and by mid-November 2021 the number was as high as 75% and slowly increased to 78% by early January 2022. Administration of booster (third) doses started in September 2021, with an uptake of about 28% by January 4, 2022. While such high rates of full vaccination could explain the minimal rise in daily cases seen from August to September, the rise in cases could more parsimonious be explained by a shift in monitoring and sampling resulting from back-to-work and school policies requiring increased systematic testing of asymptomatic unvaccinated individuals (Government of Ontario Ministry of Health, 2021). It is notable that analyses justifying vaccine mandates conducted by the Ontario Science Table were conducted within this time window and were likely heavily influenced by the differential testing policies. It should be noted that daily case counts began to sharply increase with the drop in temperature in November and December 2021, similar to the seasonal shifts in respiratory disease observed in the previous year and seemingly at variance with increasing levels of vaccination for the overall population. It is precisely these sorts of outcomes that led to claims, in late August and early September, that the unvaccinated were driving the bulk of new infections and threatening ICU capacities and that vaccine passports were required to limit the unvaccinated individuals from entering public spaces to halt further disease spread.
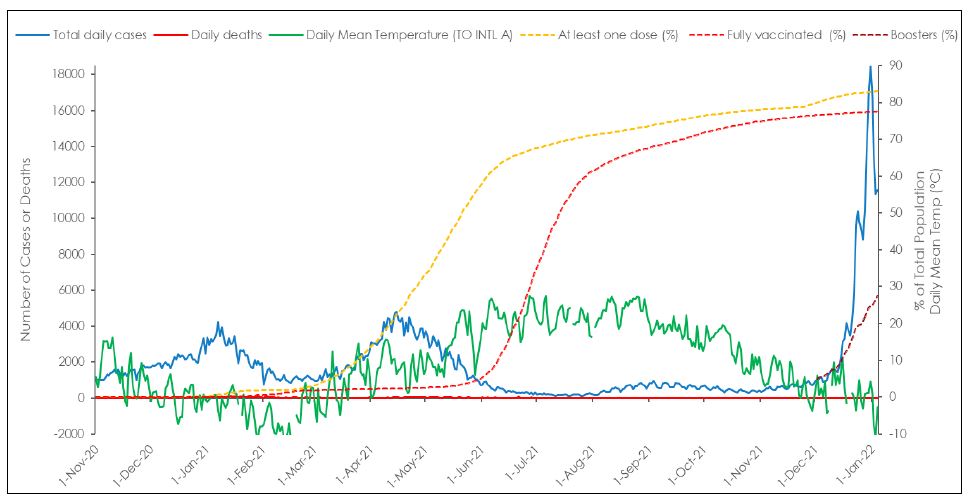
In Figure 2A, daily COVID-19 case counts by vaccination status are plotted from Aug 8, 2021 to January 4, 2022. These data also lead to reports, as cited above, that this was a pandemic of the unvaccinated. However, when cases in the unvaccinated were compared to those who had received the vaccines, the differences in case counts and rates were low and steady leading up to early October 2021, at which point case counts in the two groups began to overlap; by early December 2021, a surge in daily cases in the fully vaccinated typical of the infectious peaks of earlier waves was clearly apparent. By early January 2022, at the peak of the time period studied, the number of cases in the vaccinated were 6- fold higher than in unvaccinated.
It is important to note that unvaccinated individuals represent 20% of the population and that, children 11 years or younger, representing 15% of the population, are the largest demographic in the unvaccinated group. As COVID-19 vaccines were approved in Canada on November 19, 2021 for children 5 – 11 years old for most of the time period studied, this group was then ineligible for vaccination (Government of Canada, 2021b).
As case counts were 6-fold higher in the fully vaccinated group compared to the unvaccinated group by mid to late December 2021, the Ontario data does not provide compelling support for policy decisions recommending the vaccination of children younger than 11 years. At that time, the proportion of cases in the fully vaccinated had surpassed those of unvaccinated (7-day average: 87.89 per 100,000 vs 66.15 per 100,000, respectively), reflecting a higher degree of infectivity (Figure 2B). As currently the vast majority of the cases are estimated to be caused by the new Omicron variant, for the week of December 12th-18th 2021, Omicron was estimated to make up 66.1% of Ontario cases, rising to an estimate of 97.5% of positive Ontario cases the week of December 26th 2021–January 1, 2022 (Public Health Ontario, 2022a). It thus seemed that the vaccines designed to combat the wild-type strain have become largely obsolete. However, a third dose (the first “booster”) of these vaccines may provide some initial protection in the face of secondary vaccine failure from the initial two vaccination schedule. Although increased infection rates in the vaccinated group could be due to them engaging in higher risk activities than the unvaccinated, it is also highly possible that these data show vaccine enhanced disease, a concern raised in the Pfizer Safety Report (Nevada Division of Public and Behavioral Health, 2021).
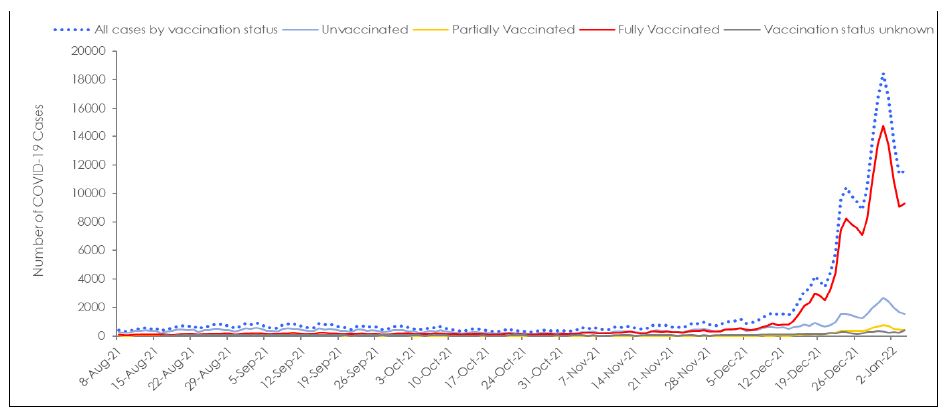
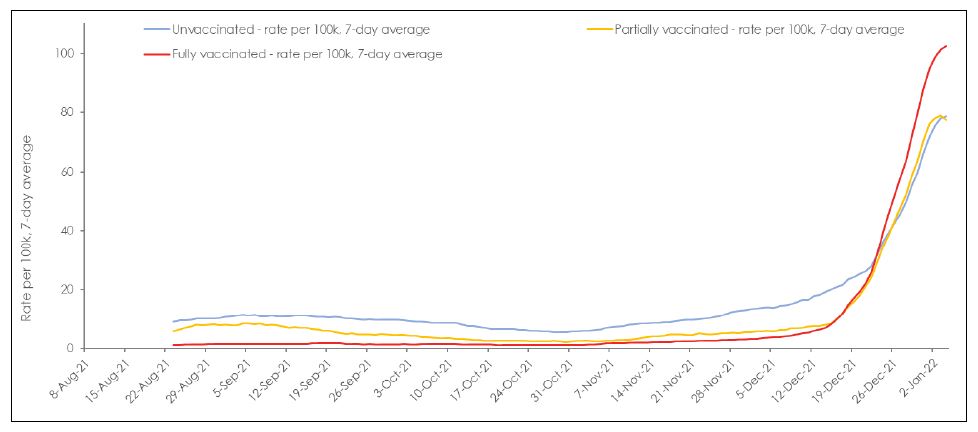
Figure 2: (A) Number of cases and (B) 7-day averaged case rates by vaccination status in Ontario from Aug 8, 2021 to Jan 4, 2022. (Government of Ontario, 2022).
Hospitalization Admissions and Death
Daily COVID-19 hospitalizations and deaths are plotted from November 1, 2020 to January 4, 2022 in Figure 3. Hospitalization admissions peaked at 200 admissions during the second wave, between 300 and 400 admissions for the third wave and were lowest for the fourth wave, and a potential fifth wave peaking at 148 cases on Jan 4, 2022. Likewise, COVID-19 deaths peaked at 100 deaths per day during the second wave, were as low as 50 deaths per day in the third wave, rarely exceeded 8 deaths per day in fourth wave, and as of January 4, 2022, deaths for the potential fifth wave have not reached more than 13 deaths per day despite the dramatic surge in cases in December 2021 that are linked to the Omicron variant.
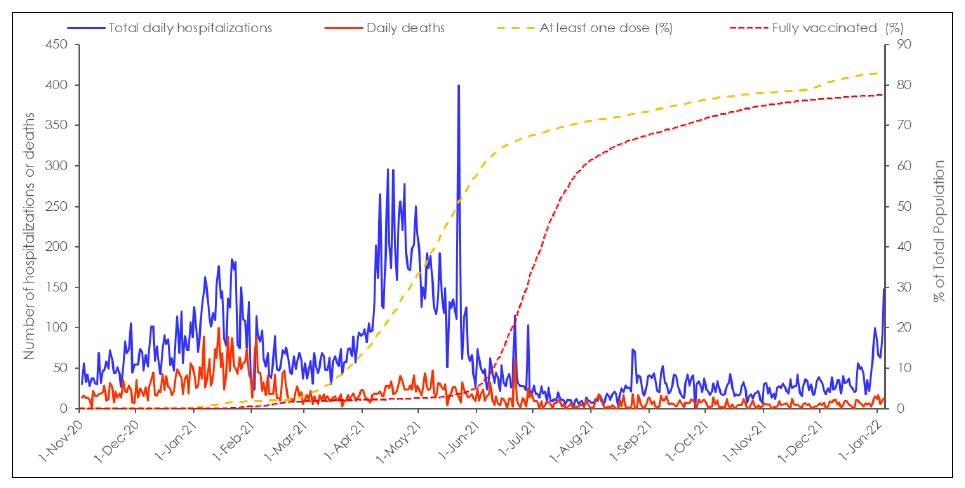
As noted above, it is possible that the lower death rates could be attributed to increasing rates of partial vaccination of high-risk populations from March to July 2021, serving to prevent more severe COVID-19 outcomes, including deaths. We find this interpretation unlikely, as only 35% of the population were vaccinated at that point and many were the elderly who are known to be immunosenescent, that is that they have a dysregulated immune system that makes it more difficult for them to develop sterilizing immunity leaving them more susceptible to infection (Crooke & Ovsyannikova et al., 2019). However, such an uncoupling could also be attributed to a lower net number of individuals who might have been at risk of death from the disease: During the first and second waves, as the majority of deaths due to COVID-19 were in long-term care facilities (Marrocco & Coke, 2021; Canadian Institute for Health Information, 2021). Death rates were high in these facilities largely because many facilities were under resourced and ill-equipped to stop aerosol transmission of SARS-CoV-2 among residents (Tang & Mao et al., 2020; Thompson & Barbu et al., 2020; World Health Organization, 2021; Dykgraaf & Matenge et al., 2021; Brown & Jones et al., 2021). These aspects, coupled with the fact that many individuals within these facilities were already at risk of severe disease (Thompson & Barbu et al., 2020; World Health Organization, 2021; Dykraaf & Matenge et al., 2021; Akhtar-Danesh & Baumann et al., 2022), that early treatment was not routinely provided (Sundraram & Nasreen et al., 2021), and that “35% of residents had no hospitalization orders and 80% had do not resuscitate” orders in place, made outbreaks in these facilities more likely to be fatal (Canadian Institute for Health Information, 2021; Morrocco & Coke, 2021).
By the beginning of the third wave many of those who were at risk of death from COVID-19 had either developed immunity or had died, limiting the number of those vulnerable to infection. While many vulnerable residents died due COVID-19 infections, others died due to failure to thrive from being isolated for a full year. Another contingent may have died due to vaccine-related injury (Wyller & Kittang et al., 2021). In this regard, it is likely relevant that a CDC report using the VAERS system that was published in early 2021 reported that 69% of all reported possible vaccine deaths were in people living in long-term care facilities and occurred a median of 2 days after vaccination (Gee & Marquez et al., 2021).
Hospitalizations and ICU due to COVID-19 by Vaccination Status
Total in hospital and ICU cases presumed to be due to COVID-19 vaccination status were plotted from Aug 8, 2021 to January 4, 2022 (Figure 4). The number of patients in hospital peaked at 526 during the early part of the time period studied, but by January 4, 2022 the number COVID-19 hospitalizations had reached up to 2,000. Although it had increased by at least 3-fold, it remained considerably below the 21,000 total number of acute care beds available in Ontario (Ontario Hospital Association, 2021). The number of people in ICU remained low and did not surpass more than 200 beds during the time period studied and thus did not come close to approaching the ICU capacity for Ontario of 2,343 beds (Government of Ontario, 2021). Although there were more unvaccinated compared to vaccinated people in the ICU throughout the initial time period studied, the number of admissions in the vaccinated rose progressively, to the point that on January 4, 2022, the number of vaccinated in ICU was very similar to that of unvaccinated (N= 109 vs 100) with ICU admissions still on the rise.
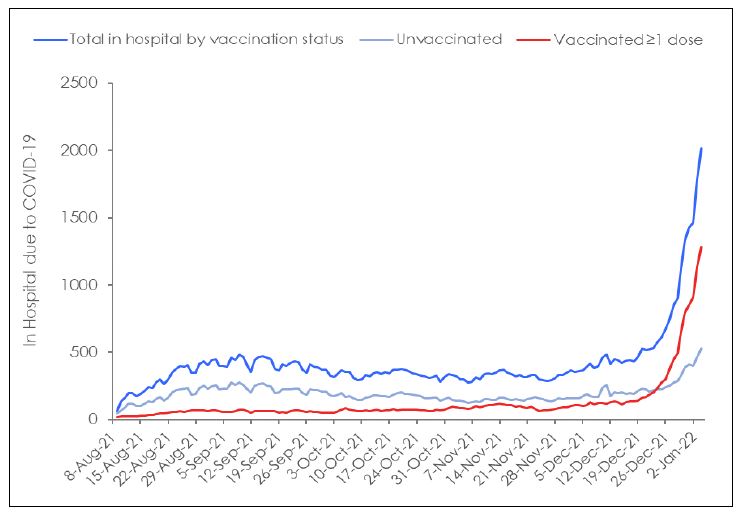
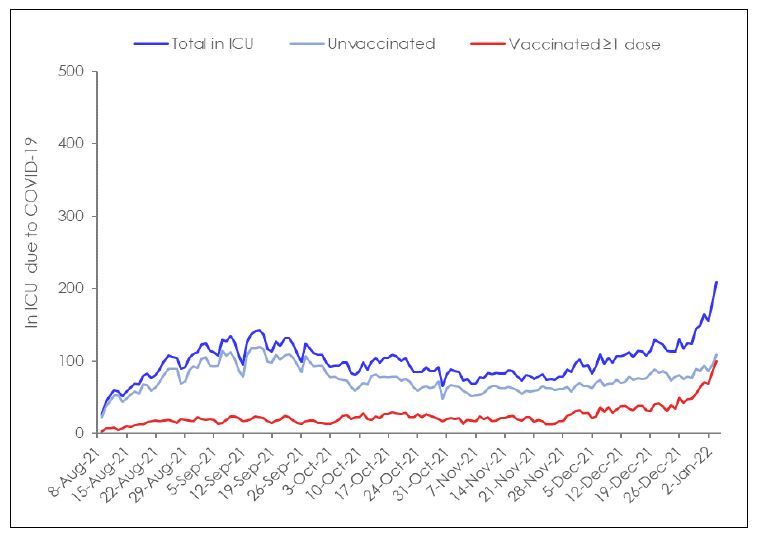
Figure 4: COVID-19 (A) Total hospitalized and (B) in ICU due to COVID-19 by vaccination status in Ontario from August 8, 2021 to Jan 4, 2022. (Government of Ontario, 2022)
The application of vaccines, as noted above, may have had a role in conferring some protection from severe disease and death from August through to the end of October 2021. However, such an interpretation does not explain why counts among the vaccinated and unvaccinated were comparably low even with counts only increasing in early November. An alternative, and to us a more likely explanation is that that differences seen in this time period are instead reflective of changes in vaccination policies requiring systematic testing of asymptomatic unvaccinated individuals. Such differences would be particularly apparent in settings such as hospitals where patients are closely monitored and may have heavily influenced the number of counts seen in this population. Additionally, as COVID-19 is transmitted in shared community living situations such as hospitals, events among the unvaccinated may be a by-product of hospital admission status. In fact, the ministry of health recently indicated that almost half of the COVID-19 hospitalizations were people who were admitted to the hospital with another condition and tested positive with COVID-19 rather than people in the hospital due to COVID-19 (Public Health Ontario, 2022c). The consistently low and comparable rates among the vaccinated and unvaccinated may strongly reflect differences in monitoring and sampling more than differences in actual infectivity.
Another notable observation from these data is that despite surges in total COVID-19 cases, the total number people in hospital and ICU remained relatively low, indicating that the COVID-19 variants are either less virulent, or that a clinically meaningful population level of vaccine and natural-induced immunity has now been achieved, or that there are fewer people at risk of severe disease either due to death or immunity.
Concerns Regarding the Safety of COVID-19 Vaccines and Current Vaccine Policies
The most significant limitation of retrospective analyses of realworld COVID-19 events is that they fail to weigh the benefits of vaccination against potential risks of vaccination. Randomized placebo controlled trials are the most reliable source of safety and efficacy data available. The 6-month data from the Pfizer phase III trial (Table 1) showed that receipt of the BNT1626b2 vaccine increased both the absolute (17.9%) and relative (299.7%) risk of experiencing a vaccine-related adverse event in fully vaccinated adults (Thomas & Moreira et al., 2021). This study also reported relative (74.6%) and absolute (0.5%) increases in severe adverse events, events that affect daily activity, require medical intervention, or require hospitalization. Likewise, there were also relative (9.5%) and absolute (0.05%) risk increases in serious adverse event, events that require hospitalization, were life-threatening, or resulted in persistent disability or death.
When deaths were considered for both the blinded and open-label periods of the study, there was an increase in deaths on the vaccine arm compared to the placebo arm (20 deaths vs 14 deaths), many of which were cardiovascular in nature (9 deaths vs 5 deaths). Although the Pfizer phase trials reported relative risk reductions in symptomatic COVID-19 cases (90.9%) and severe COVID-19 cases (95.7%) with BNT1626b2 compared to placebo, the absolute risk reductions were modest (mild to moderate 3.7% and severe 0.1%) and lower than the absolute risk increases in adverse events (any 17.8%, severe 0.05%). When the absolute benefit of the vaccine is compared to the absolute risk, it appears that the vaccine, and by extension current vaccine policy, may be doing more harm than good.
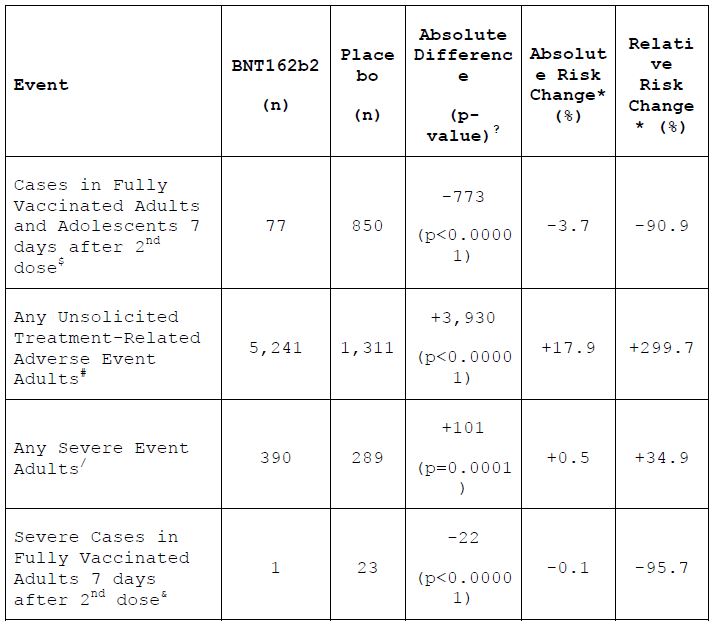
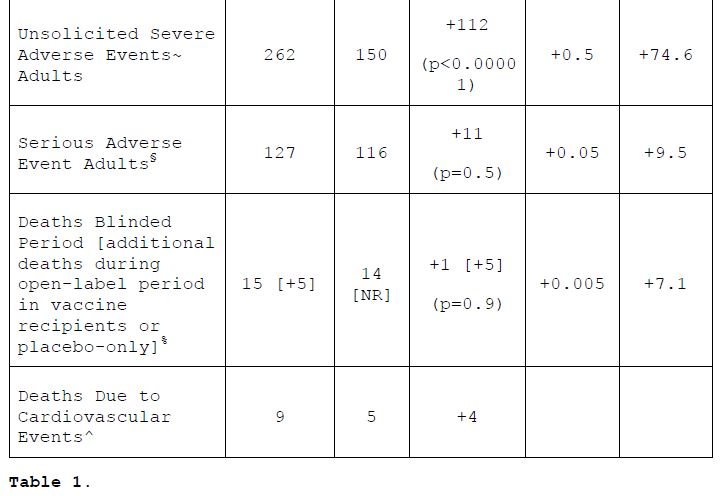
¥ For the purpose of this table and according to the terminology used in the study report, adult and adolescent populations are defined as ≥16 years old and 12-15 years old, respectively.
? Significance figures (p-values) estimated using chi-square calculator available at https://www.socscistatistics.com/tests/chisquare. P-values are without the Yates correction. This procedure was applied following the framework used by Classen (2021) in his analysis of “All Cause Severe Morbidity” based on data from the initial reports of the vaccine Phase III trials. (source 15)
* Authors estimated vaccine efficacy using total surveillance time as denominator, however, as this value was unavailable for all the events analyzed, our calculations used the common statistical definition, i.e., number of events relative to total number of eligible patients for each event analysis reported (source 16) similar to previous analyses of this nature. (sources 15,17)
$ ≥7 Days after dose 2 among participants without evidence of previous infection.
#Adverse events reported outside of the reactogenicity subgroup and assessed by the investigator as related to investigational product.
In calculations combining efficacy and safety events, the number of patients randomized that received any dose of vaccine or placebo was used as the study population in the statistical calculations, following the framework used by Classen(2021) in his analysis of “All Cause Severe Morbidity”. Differences in the total (event-incident) population (randomized vs efficacy vs safety) used as denominator are relatively small and are expected to have minimal impact on the relative differences between groups. Without access to individual patient data, these calculations were performed under the assumption that efficacy and safety events were non-overlapping.
& ≥7 Days after dose 2; confirmed severe COVID-19 defined as PCR-positivity and “presence of at least 1 of the following: • Clinical signs at rest indicative of severe systemic illness (RR ≥30 breaths per minute, HR ≥125 beats per minute, SpO2 ≤93% on room air at sea level, or PaO2/FiO2 <300 mm Hg); • Respiratory failure (defined as needing high-flow oxygen, noninvasive ventilation, mechanical ventilation, or ECMO); • Evidence of shock (SBP <90 mm Hg, DBP <60 mm Hg, or requiring vasopressors); • Significant acute renal, hepatic, or neurologic dysfunction; • Admission to an ICU; • Death.”
~ Severe (grade ≥3) adverse events were generally defined as those that interfere significantly with participant’s usual function, those that affect daily living or require medical care; grade 4 events were generally defined as those that required emergency room visit or hospitalization.
§ Serious adverse events were defined as any untoward medical occurrence that, at any dose: a. Results in death; b. Is life threatening; c. Requires inpatient hospitalization or prolongation of existing hospitalization; d. Results in persistent disability/incapacity.
% Deaths during the open-label period were reported only in vaccine recipients, 3 participants in the BNT162b2 group and 2 in the original placebo group who received BNT162b2 after unblinding.
^Those with reported cause of death due to: aortic rupture, arteriosclerosis, cardiac arrest, cardiac failure congestive, cardiorespiratory arrest, hypertensive heart disease, or myocardial infarction.
Although there is a sophisticated system in place for monitoring and tracking COVID-19 events, COVID-19 Ontario relies on a national passive surveillance system to monitor adverse events. This system launched decades ago, is burdensome, heavily audited, and provides no financial compensation to clinicians for the considerable time required to complete the reports. Moreover, assertions on the part of public health officials claiming vaccine safety may make clinicians and vaccine recipients alike less likely to attribute an injury to the vaccine and thus make them less likely to report adverse events.
The phase III trials report a solicited adverse event rate of up to 78% and a severe adverse event rate of 5% within 7 days of a given dose (Figure 5). However, Health Canada’s passive surveillance system reports an event rate of 0.1% for vaccine recipients (Government of Canada, 2021a). This indicates that the passive reporting system is largely inadequate for monitoring COVID-19 vaccine safety and that there are likely a significant number of Canadians experiencing adverse effects from these vaccines that are not being captured by this system and that are not being factored into our policy decision-making.
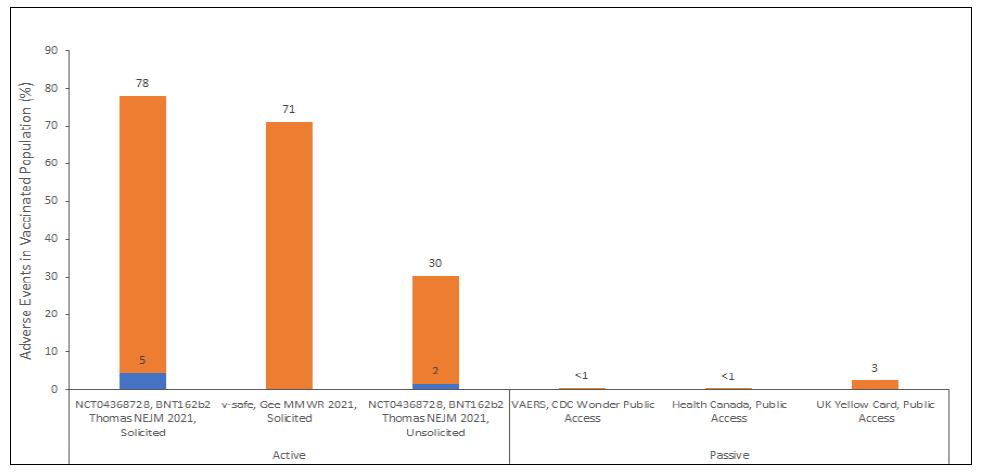
Figure 5: The rate of solicited and unsolicited adverse events in active surveillance studies of COVID-19 vaccines (NCT04368728 and v-safe) and proportion of adverse events following COVID-19 vaccination reported to passive surveillance systems (VAERS (U.S. Department of Health and Human Services, 2021), Health Canada (Government of Canada, 2021a) and UK Yellow Card (UK Government, 2021)) relative to total vaccinated (≥1 dose) population.
Conclusions
Health officials claim that the unvaccinated are driving infection rates and that mandates are necessary to preserve hospital capacity. However, a careful inspection of the data indicates not only were these policies are based low quality evidence that was highly subject to bias, the actual data demonstrated the opposite trend. Although there was a slight increase in cases among the unvaccinated from August through to mid-October 2021, reflective on increased testing rates, by early December 2021 the majority of cases were among the vaccinated. Moreover, this surge in cases resulted in a higher proportion of vaccinated compared to unvaccinated individuals in the hospital or ICU admissions despite vaccine mandates and high vaccine uptake. Finally, the policy did not adequately weigh the benefits of vaccination against potential risks, which outweigh (adverse events, ARI of 17.9% and 0.5%) any benefits derived from the vaccines (symptomatic cases, ARR of 3.7% and 0.1%) (Thomas & Moreira et al., 2021).
Optimal care should be characterized by, scientific discourse and inquiry, reliance on the highest levels of evidence, individualized care, and informed consent (Chow & Gallo et al., 2018; Madden & Bhandari, 2020; Shah & Turrin et al., 2021. As COVID-19 is no longer a risk to the population at large due to the arrival of less virulent strains like Omicron, or the latest B.2 variant, and the widespread population-based immunity, it is time to abandon these outdated vaccines and mandates and return to good medical practice and established standards of care.
Conflicts of Interest
DMc, SP, and CAS are all members of the Science and Medical Advisory Committee for the Canada Covid Care Alliance. No other conflicts of interest are declared.
Acknowledgements
The authors thank Suresh Bairwa for help with formatting.
References
Akhtar-Danesh, N., Baumann, A., Crea-Arsenio, M., Antonipillai, V. (2022). COVID-19 excess mortality among long-term care residents in Ontario, Canada. PLoS One 2022;17:e0262807.
Brown, CM., Vostok, J., Johnson, H., et al. (2021, July). Outbreak of SARS-CoV-2 infections, including COVID-19 vaccine breakthrough infections, associated with large public gatherings—Barnstable County, Massachusetts, July 2021. Morb Mortal Weekly Rep 2021;70:1059.
Brown, KA., Jones, A., Daneman, N., et al. (2021). Association between nursing home crowding and COVID-19 infection and mortality in Ontario, Canada. JAMA Intern Med 2021;181:229-36.
Burdin, N., Handy, LK., Plotkin, SA. (2017). What is wrong with pertussis vaccine immunity? The problem of waning effectiveness of pertussis vaccines. Cold Spring Harb Perspect Biol 2017;9.
Canadian Blood Services COVID-19 Seroprevalence Brief Report 19A: February 1-15, 2022 Survey. https://www.covid19immunitytaskforce.ca/wpcontent/uploads/2022/04/covid-19-report-feb-2022-march-2022.pdf
Canadian Institute for Health Information. (2021). The impact of COVID-19 on long-term care in Canada: Focus on the first 6 months. CIHI Ottawa, ON, Canada; 2021.
CBC News. (2021, August 17). Ontario announces mandatory vaccine plans for health, education workers; 3rd doses for some. CBC News. Retrieved January 29, 2022, from
https://www.cbc.ca/news/canada/toronto/ontario-covid-vaccines-requirement-health-care-education-1.6143378
Centers for Disease Control and Prevention. (2021, October 29). Frequently asked questions about Coronavirus (COVID-19) for laboratories – Interpreting results of diagnostic tests. Retrieved October 29, 2021, from
https://www.cdc.gov/coronavirus/2019-ncov/lab/faqs.html#Interpreting-Results-of-Diagnostic-Tests
Chow, N., Gallo, L., Busse, JW. (2018). Evidence-based medicine and precision medicine: Complementary approaches to clinical decision-making. Precision Clinical Medicine 2018; 1:60-4.
City of Toronto. (2022). COVID-19: Epidemiological summary of cases – Which COVID-19 cases required medical attention? Retrieved January 29, 2022, from https://www.toronto.ca/home/COVID-19/COVID-19-pandemicdata/COVID-19-epidemiological-summary-of-cases-data/
Cohen, KW., Linderman, SL., Moodie, Z., et al. (2021). Longitudinal analysis shows durable and broad immune memory after SARS-CoV-2 infection with persisting antibody responses and memory B and T cells. Cell Rep Med 2021;2:100354.
Collie, S., Champion, J., Moultrie, H., Bekker, L-G., Gray, G. (2021). Effectiveness of BNT162b2 vaccine against omicron variant in South Africa. N Engl J Med 2021.
Connell, AR., Connell, J., Leahy, TR., Hassan, J. (2020). Mumps outbreaks in vaccinated populations—Is it time to re-assess the clinical efficacy of vaccines? Front Immunol 2020;11.
Crooke, SN., Ovsyannikova, IG., Poland, GA., Kennedy, RB. (2019). Immunosenescence and human vaccine immune responses. Immun Ageing 2019;16:25. Dejnirattisai, W., Shaw, RH., Supasa, P., et al. (2021). Reduced neutralisation of SARS-COV-2 Omicron-B.1.1.529 variant by postimmunisation serum. medRxiv 2021:2021.12.10.21267534.
Duintjer Tebbens, RJ., Pallansch, MA., Chumakov, KM., et al. (2013). Expert review on poliovirus immunity and transmission. Risk Anal 2013;33:544-605.
Dunham, J. (2021, August 19). Unvaccinated? Here are some of the things that are off-limits to you in Canada. CTV News. Retrieved January 29, 2022, from https://www.ctvnews.ca/health/coronavirus/unvaccinated-here-aresome-of-the-things-that-are-off-limits-to-you-in-canada-1.5553655
Dykgraaf, SH., Matenge, S., Desborough, J., et al. (2021). Protecting nursing homes and long-term care facilities from COVID-19: A rapid review of international evidence. J Am Med Dir Assoc 2021;22:1969-88.
Edmonson, MB., Addiss, DG., McPherson, JT., Berg, JL., Circo, SR., Davis, JP. (1990). Mild measles and secondary vaccine failure during a sustained outbreak in a highly vaccinated population. JAMA 1990;263:2467-71.
Focosi, D., Maggi, F., Casadevall, A. (2022). Mucosal vaccines, sterilizing immunity, and the future of SARS-CoV-2 virulence. Viruses 2022;14:187.
Gee, J., Marquez, P., Su, J., et al. (2021). First month of COVID-19 vaccine safety monitoring – United States, December 14, 2020-January 13, 2021. MMWR Morb Mortal Wkly Rep 2021;70:283-8.
Goodfield, K. (2021, February 2). Ontario records 745 new COVID-19 cases, 14 deaths while citing data issue. CTV News. Retrieved January 29, 2022 from https://toronto.ctvnews.ca/ontariorecords-745-new-COVID-19-cases-14-deaths-while-citing-dataissue-1.5292064
Government of Canada. (2021a). Health Canada. Reported side effects following COVID-19 vaccination in Canada. Retrieved January 29, 2022, from https://health-infobase.canada.ca/COVID-19/vaccine-safety/
Government of Canada. (2021b). Health Canada authorizes use of Comirnaty (the Pfizer-Biontech COVID-19 Vaccine) in children 5 to 11 years of age. Retrieved January 29, 2022, from https://www.canada.ca/en/health-canada/news/2021/11/healthcanada-authorizes-use-of-comirnaty-the-pfizer-biontech-COVID-19-vaccine-in-children-5-to-11-years-of-age.html
Government of Canada. (2022). Historical weather and climate data for Toronto International A Station. Retrieved January 29, 2022, from https://climate.weather.gc.ca/climate_data/daily_data_e.html?StationID=51459
Government of Ontario. (2021). COVID-19: Hospitalizations. ontario.ca, 2021. Retrieved January 29, 2022, from https://COVID-19.ontario.ca/data/hospitalizations#hospitalizationsByICUBed
Government of Ontario. (2022). Ontario Data Catalogue – COVID-19 vaccine data in Ontario. Retrieved January 29, 2022, from https://data.ontario.ca/dataset/COVID-19-vaccine-data-in-ontario
Government of Ontario Ministry of Health. (2021). Management of cases and contacts of COVID-19 in Ontario August 11, 2021 (version 13.0). Retrieved January 29, 2022, from https://www.health.gov.on.ca/en/pro/programs/publichealth/coronavirus/docs/contact_mngmt/management_cases_contacts.pdf
Heffernan, JM., Keeling, MJ. (2008). An in-host model of acute infection: measles as a case study. Theor Popul Biol
2008;73:134-47.
Hellerstein, M. (2020). What are the roles of antibodies versus a durable, high quality T-cell response in protective immunity against SARS-CoV-2? Vaccine X 2020;6:100076.
Herhalt, C. (2021, September 5). Unvaccinated Ontario teachers must submit to twice weekly COVID-19 testing. CTV News. Retrieved January 29, 2022, from https://toronto.ctvnews.ca/unvaccinated-ontario-teachers-mustsubmit-to-twice-weekly-COVID-19-testing-1.5574333
Hogan, A., Winskill, P., Watson, O., et al. (2020, September 25). Report 33: modelling the allocation and impact of a COVID-19 vaccine. Imperial College of London. Retrieved January 29, 2022, from https://www.imperial.ac.uk/mrc-global-infectiousdisease-analysis/COVID-19/report-33-vaccine/
Kompaniyets, L., Goodman, AB., Belay, B., et al. (2021). Body mass index and risk for COVID-19–related hospitalization, intensive care unit admission, invasive mechanical ventilation, and death—United States, March–December 2020. Morb Mortal Weekly Rep 2021;70:355.
Keegan, LT., Truelove, S., Lessler, J. (2021). Analysis of vaccine effectiveness against COVID-19 and the emergence of delta and other variants of concern in Utah. JAMA Network Open 2021;4:e2140906-e.
Keeling, M., Tildesley, M., House, T., Danon, L. (2013). The mathematics of vaccination. Math Today 2013;49:40-3.
Kompaniyets, L., Pennington, AF., Goodman, AB., et al. (2021). Underlying medical conditions and severe illness among 540,667 adults hospitalized with COVID-19, March 2020-March 2021. Prev Chronic Dis 2021;18:E66.
Koopman, JS., Henry, CJ., Park, JH., Eisenberg, MC., Ionides, EL., Eisenberg, JN. (2017). Dynamics affecting the risk of silent circulation when oral polio vaccination is stopped. Epidemics 2017;20:21-36.
Lewnard, JA., Grad, YH. (2018). Vaccine waning and mumps reemergence in the United States. Sci Transl Med 2018;10.
Lopez Bernal, J., Andrews, N., Gower, C., et al. (2021). Effectiveness of COVID-19 vaccines against the B.1.617.2 (Delta) Variant. N Engl J Med 2021;385:585-94.
Madden, K., Bhandari, M. (2020). Can evidence-based medicine and personalized medicine coexist? Personalized Hip and Knee Joint Replacement 2020:1-5.
Mann, CJ. (2003). Observational research methods. Research design II: cohort, cross sectional, and case-control studies. Emerg Med J 2003;20:54-60.
Mathias, RG., Meekison, WG., Arcand, TA., Schechter, MT. (1989). The role of secondary vaccine failures in measles outbreaks. Am J Public Health 1989;79:475-8.
Mina, MJ., Peto, TE., García-Fiñana, M., Semple, MG., Buchan, IE. (2021). Clarifying the evidence on SARS-CoV-2 antigen rapid tests in public health responses to COVID-19. The Lancet 2021;397:1425-7.
Misra, S. (2012). Randomized double blind placebo control studies, the “Gold Standard” in intervention based studies. Indian J Sex Transm Dis AIDS 2012;33:131-4.
Morawska, L., Tang, JW., Bahnfleth, W., et al. (2020). How can airborne transmission of COVID-19 indoors be minimised? Environ Int 2020;142:105832.
Morgan, G. (2021, October 18). Most COVID vaccinated Canadians mistrustful of the unvaccinated and medical exemptions: poll. National Post. Retrieved January 29, 2022, from https://nationalpost.com/news/canada/most-COVID-vaccinatedcanadians-mistrustful-of-the-unvaccinated-and-medicalexemptions-poll
Marrocco, FN., Coke, A., Kitts, J. (2021, April 30). Ontario’s long-term care COVID-19 commission final report. Retrieved January 29, 2022, from http://www.ltccommissioncommissionsld.ca/report/pdf/20210623_LTCC_AODA_EN.pdf
Ontario Hospital Association. (2021). Fact sheet on hospital capacity. Retrieved January 29, 2022, from https://www.oha.com/Bulletins/OHA%20Fact%20Sheet%20on%20Hospital%20Capacity%20and%20Projections%20Jan%2015%202021.pdf
Ontario Ministry of Health. (2021). COVID-19 fully vaccinated and previously positive individuals: Case, contact and outbreak management interim guidance. Retrieved January 29, 2022, from https://www.health.gov.on.ca/en/pro/programs/publichealth/coronavirus/docs/contact_mngmt/COVID-19_fully_vaccinated_interim_guidance.pdf
Nevada Division of Public and Behavioral Health. (2021, December 3). Nevada Board of Health Meeting (pp. 103). Retrieved January 29, 2022, from https://dpbh.nv.gov/uploadedFiles/dpbhnvgov/content/Boards/BOH/Meetings/2021/Public%20Comments%20276%20to%20294.pdf
Parsons, P. (2022) Health expert urges caution after bloodtesting firm claims ‘pandemic is over’ in Albert hamlet. https://www.cbc.ca/news/canada/edmonton/private-covid-19-antibody-tests-la-crete-alberta-1.6307357
Phillips, N. (2021). The coronavirus is here to stay-here’s what that means. Nature 2021;590:382-4.
Pollard, AJ., Bijker, EM. (2021). A guide to vaccinology: from basic principles to new developments. Nature Reviews Immunology 2021;21:83-100.
Pringle J. (2021, October 22). Unvaccinated Ottawa police employees must undergo testing every three days under COVID-19 vaccine policy. CTV News. Retrieved January 29, 2022, from https://ottawa.ctvnews.ca/unvaccinated-ottawa-police-employeesmust-undergo-testing-every-three-days-under-COVID-19-vaccinepolicy-1.5634864
Public Health Ontario. (2020). An overview of cycle threshold values and their role in SARS-CoV-2 real-time PCR test interpretation. Retrieved January 29, 2022, from https://www.publichealthontario.ca/-/media/documents/ncov/main/2020/09/cycle-threshold-values-sarscov2-pcr.pdf?la=en
Public Health Ontario. (2021a). Explained: COVID-19 PCR testing and cycle thresholds. Retrieved January 29, 2022, from https://www.publichealthontario.ca/en/about/blog/2021/explained-COVID19-pcr-testing-and-cycle-thresholds
Public Health Ontario. (2021b). Trends of COVID-19 incidence in Ontario. Retrieved January 29, 2022, from https://www.publichealthontario.ca/-/media/documents/ncov/epi/COVID-19-epi-trends-incidenceontario.pdf?sc_lang=en
Public Health Ontario. (2022a). Sars-Cov-2 whole genome sequencing in Ontario. Retrieved January 29, 2022, from https://www.publichealthontario.ca/-/media/documents/ncov/epi/COVID-19-sars-cov2-whole-genomesequencing-epi-summary.pdf?sc_lang=en
Public Health Ontario. (2022b). Ontario COVID-19 data tool. Retrieved January 29, 2022, from https://www.publichealthontario.ca/en/data-andanalysis/infectious-disease/COVID-19-data-surveillance/COVID-19-data-tool?tab=summary
Public Health Ontario. (2022c). Confirmed cases of COVID-19 following vaccination in Ontario: December 14, 2020 to January 16, 2022. Retrieved January 29, 2022, from https://www.publichealthontario.ca/-/media/documents/ncov/epi/COVID-19-epi-confirmed-cases-postvaccination.pdf?sc_lang=en
Ranstam, J. (2019). Hypothesis-generating and confirmatory studies, Bonferroni correction, and pre-specification of trial endpoints. Acta Orthop 2019;90:297-.
Read, AF., Mackinnon, MJ. (2009). Pathogen evolution in a vaccinated world. Evolution in health and disease 2008;2:139-52.
Read, AF., Baigent, SJ., Powers, C., et al. (2015). Imperfect vaccination can enhance the transmission of highly virulent pathogens. PLoS Biol 2015;13:e1002198.
Reis, BY., Barda, N., Leshchinsky, M., et al. (2021). Effectiveness of BNT162b2 vaccine against Delta variant in adolescents. N Engl J Med 2021;385:2101-3.
Science Table – COVID-19 Advisory for Ontario. (2021, September 28). Update on COVID-19 projections – Science advisory and modelling consensus tables. Retrieved January 29, 2022, from
https://COVID19-sciencetable.ca/wpcontent/uploads/2021/09/Update-on-COVID-19-Projections_2021.09.28_English-2.pdf
Scott, J., Richterman, A., Cevik, M. (2021). COVID-19 vaccination: evidence of waning immunity is overstated. BMJ 2021;374:n2320.
Shah, P., Thornton, I., Turrin, D., & Hipskind, J. E. (2021). Informed Consent. In StatPearls. StatPearls Publishing.
Singanayagam, A., Hakki, S., Dunning, J., et al. (2021). Community transmission and viral load kinetics of the SARS-CoV-2 delta (B.1.617.2) variant in vaccinated and unvaccinated individuals in the UK: a prospective, longitudinal, cohort study. Lancet Infect Dis 2021;22:183-95.
Sundaram, M., Nasreen, S., Calzavara, A., et al. (2021). Background rates of all-cause mortality, hospitalizations, and emergency department visits among nursing home residents in Ontario, Canada to inform COVID-19 vaccine safety assessments. Vaccine 2021;39:5265-70.
Talari, K., Goyal, M. (2020). Retrospective studies – utility and caveats. J R Coll Physicians Edinb 2020;50:398-402.
Tang, S., Mao, Y., Jones, RM., et al. (2020). Aerosol transmission of SARS-CoV-2? Evidence, prevention and control. Environ Int 2020;144:106039-.
Thiruvengadam, R., Awasthi, A., Medigeshi, G., et al. (2021). Effectiveness of ChAdOx1 nCoV-19 vaccine against SARS-CoV-2 infection during the delta (B.1.617.2) variant surge in India: a test-negative, case-control study and a mechanistic study of post-vaccination immune responses. Lancet Infect Dis 2021.
Thomas, SJ., Moreira, ED., Kitchin, N., et al. (2021). Safety and efficacy of the BNT162b2 mRNA COVID-19 vaccine through 6 months. N Engl J Med 2021;385:1761-73.
Thompson, D-C., Barbu, M-G., Beiu, C., et al. (2021). The impact of COVID-19 pandemic on long-term care facilities worldwide: An overview on international issues. BioMed Research International 2020;2020:8870249.
Torgovnick, J. (2021). Effectiveness of COVID-19 vaccines against the B.1.617.2 (delta) variant. N Engl J Med 2021.
UK Government – Medicines & Healthcare Products Regulatory Agency. (2021). Coronavirus vaccine – weekly summary of Yellow Card reporting. Retrieved January 29, 2022, from https://www.gov.uk/government/publications/coronavirus-COVID-19-vaccine-adverse-reactions/coronavirus-vaccine-summary-of-yellowcard-reporting
U.S. Department of Health and Human Services (HHS). (2021). Vaccine Adverse Event Reporting System – VAERS Data. Retrieved January 29, 2022, from https://vaers.hhs.gov/data.html
Vygen, S., Fischer, A., Meurice, L., et al. (2016). Waning immunity against mumps in vaccinated young adults, France 2013. Euro Surveill 2016;21:30156.
Woolf, SH., Chapman, DA., Lee, JH. (2021). COVID-19 as the leading cause of death in the United States. JAMA 2021;325:123-4.
World Health Organization. (2020, December 31). Preparedness WTE. Coronavirus disease (COVID-19): Serology, antibodies and immunity. Retrieved January 29, 2022, from
https://www.who.int/news-room/questions-andanswers/item/coronavirus-disease-COVID-19-serology
World Health Organization. (2021). Infection prevention and control guidance for long-term care facilities in the context of COVID-19: interim guidance, 8 January 2021. Retrieved January 29, 2022, from https://apps.who.int/iris/rest/bitstreams/1326056/retrieve
Wyller, TB., Kittang, BR., Ranhoff, AH., Harg, P., Myrstad, M. (2021). Nursing home deaths after COVID-19 vaccination. Tidsskrift for Den norske legeforening. Tidsskrift for Praktisk Medicin, ny Raekke. 2021 May;141. DOI: 10.4045/tidsskr.21.0383.PMID: 34018389.
Yewdell, JW. (2021). Individuals cannot rely on COVID-19 herd immunity: Durable immunity to viral disease is limited to viruses with obligate viremic spread. PLoS Pathog 2021;17:e1009509. https://doi.org/10.1371/journal.ppat.1009509
Young, L. (2021, September 29). Unvaccinated 60 times more likely to end up in ICU with COVID-19, Ontario data shows. GlobalNews. https://globalnews.ca/news/8230051/COVID-vaccinehospitalization-risk-ontario/
Zhang, Z., Chen, M., Ma, R., Pan, J., Suo, L., Lu, L. (2018). Outbreak of measles among persons with secondary vaccine failure, China, 2018. Hum Vaccin Immunother 2020;16:358-62. https://neuro.unboundmedicine.com/medline/citation/31487215/Outbreak_of_measles_among_persons_with_secondary_vaccine_failure_China_2018_
This information was shared from an email.
Fiction Disguised as Science to Promote Hatred
Disinformation Must Be Called Out
Dr. Byram W. Bridle Apr 26, 2022 |
If you only have a few minutes, jump to the section below entitled “Proof that the Paper by Fisman, et al. Should be Retracted Immediately”.
The legacy media has been like pigs at a trough today and yesterday with the publication of an atrocious ‘peer-reviewed’ ‘scientific’ article by Dr. David Fisman, Dr. Ashleigh Tuite, and a graduate student. After all, with raw public health data unable to support the only COVID-19 narrative that has been deemed acceptable, fresh fuel was apparently needed. Lots of media outlets have been reporting on this study; one of them being CTV News. Here is the headline for their article:
“Being with unvaccinated people increases COVID-19 risk for those who are vaccinated: modelling study”
This kind of messaging will only fuel hatred and segregation and the potential development of harmful policies. And it is all in the name of bad science. As a researcher who has published and reviewed many scientific papers, I can tell you that the article by Fisman, et al. is the worst one that I have ever seen. The ‘peer reviewers’ of this article should be ashamed of themselves for allowing this to be published, and the editor even more so. If the Canadian Medical Association Journal does not promptly retract this article, they will have made themselves an embarrassment among scientific publishers.
This paper by Fisman, et al. is only thinly veiled hate speech under the guise of science. Before I walk you through the numerous massive errors in this paper, let me first show you one example of the messaging regarding the impact and relevance of the paper.
Fisman was quoted by CTV News as saying “We thought what was missing from that conversation was, what are the rights of vaccinated people to be protected from unvaccinated people?”. The only reason why Fisman is trying to turn people against other people is due to the abject failure of the COVID-19 ‘vaccines’ to function like vaccines. That is to say that the purpose of a vaccine is to protect people from a pathogen; to prevent both the disease and transmission of the causative agent. Such a medical product does not require one to pit people against people. Fisman has inappropriately labelled critically thinking people as an enemy when the actual culprits are SARS-CoV-2 and overly rushed jabs of exceptionally poor quality and highly questionable safety that have been pushed as the sole solution at the expense of all others.
One of the few accurate statements in the CBC News article was this quote about Fisman where: “he acknowledges that a simple mathematical model doesn’t fully reflect the real world or the diverse factors that must be taken into account when setting public health policy”. This is an understatement if ever I saw one.
I already spend an inordinate amount of time and energy correcting misinformation and disinformation coming from scientists who should know better. As such, I am not going to conduct an exhaustive breakdown of the article by Fisman, et al. However, here are quite a few examples that make it clear that the science is flawed and the messaging not only biased, but completely incorrect…
- Minor issue: “While the ability to vaccinate to herd immunity has been held back by the increasing transmissibility of novel SARS-CoV-2 variants of concern…”. How about considering the fact that the ‘vaccines’ are so far from performing like real vaccines that they never could have been used as tools to achieve herd immunity. The authors of the paper are not immunologists and this has been an ongoing issue when discussions have turned to the immunological sub-discipline of vaccinology. They don’t seem to realize that the term ‘immunity’ in ‘herd immunity’ means protected from disease and transmission of the causative agent of that disease; otherwise herd immunity would be an unrealistic goal. To do this via vaccination, sterilizing or near-sterilizing immunity is required. The current SARS-CoV-2 vaccines don’t come close to meeting this standard. This is painfully obvious by the fact that SARS-CoV-2 has been allowed to become endemic despite most of the population having taken multiple doses. Look at how many work places and other organizations (and cruise ships, and research stations in Antarctica) have had SARS-CoV-2 run rampant through their 100% or almost 100% ‘vaccinated’ populations.
- Major issue: “antivaccine sentiment, fuelled in part by organized disinformation efforts, has resulted in suboptimal uptake of readily available vaccines”.In fact, the article by Fisman, et al. represents one of the most egregious examples of disinformation that I have seen.Most of the people that remain ‘unvaccinated’ are not ‘anti-vaccine’ by any stretch of the imagination. Due to critical thinking and following the science, they are not in support of the current crop of COVID-19 jabs. Remember, the definition of a vaccine was changed to accommodate these jabs. They are nothing like any of the historically mandated vaccines, such as those used in the childhood immunization series.People have been wary because the initial clinical trials to evaluate these ‘vaccines’ are still ongoing, literally meaning the jabs are still in the experimental phase. Other reasons for wariness include that the stated endpoint of the ongoing clinical trials is a reduction in cases of COVID-19, which they are failing to do. Hesitancy is because the placebo control arms of the clinical trials were removed (in contravention of study protocols) after a median follow-up time of only two months so no mid- to long-term safety signals can be detected via active monitoring. It is because the only safety monitoring left is via outdated and flawed passive voluntary monitoring systems that dramatically underestimate adverse events. It is because the vaccines behave in stark contrast to what was publicly stated. For example, they don’t stay at the injection site. Rather, the mRNA vaccines get distributed throughout the body (see my recent article about this). It is because serious side-effects were only unveiled after the rollout into the public, leading to things like the AstraZeneca ‘vaccine’ being deemed too dangerous for Canadian adults (many other countries detected the blood clotting problem and avoided this jab and then had to teach Canadian health authorities about this problem) and the Moderna vaccine being declared too dangerous for young males by harming their hearts.Other reasons for COVID-19 vaccine hesitancy include public health ‘experts’ disseminating disinformation like what is contained in the article by Fisman, et al. Also, many people recognize the validity of naturally acquired immunity and the massive accumulation of literature that shows it is superior in almost every way to the ‘vaccine’-induced immune responses. And there are a myriad of other reasons.I refer to this as a major issue because Fisman and his colleagues are labeling people and using subjective argumentation in an attempt to justify it. This sentence in the paper has no place in the world of objective science.
- Major problem: “Nonvaccination is expected to result in amplification of disease transmission in unvaccinated subpopulations, but the communicable nature of infectious diseases means that this also heightens risk for vaccinated populations”. Some critical considerations have been missed here. The issue is not about nonvaccinated versus vaccinated people; it is about who is immune and who is not. Fisman, et al. really need some advanced immunology training before opining about vaccines. They need to realize that immune responses to vaccination follow a bell-shaped curve in an outbred population like humans. Most people respond moderately well. A few will respond very robustly; these are the high responders. And then a minority will be low- or non-responders. These individuals will have no protection against disease. As such, showing certification of having received two or more jabs does not guarantee immunity. And then there is the reality that the human immune system can work in the absence of external ‘hand of man’ interventions. This means that our immune systems can mount protective responses to natural infections. Having lived with SARS-CoV-2 for more than two years and with the Delta and Omicron variants having ripped through the population, a vast majority of ‘unvaccinated’ people would be expected to have naturally acquired immunity. Conflating the concepts of immunity and vaccination represents a major conceptual flaw with this paper. I recommend that Fisman and his colleagues review this video that I am in full agreement with: Fauci teaches that natural infection is the best form of vaccination.
- Major issue that proved to be a fatal flaw: “We also assumed that some fraction of the unvaccinated population had immunity at baseline owing to previous infection and that a fraction of the population was vaccinated”. Initially, this seems reasonable. But then one learns that the number plugged into the simple mathematical model was only 20% of the population having naturally acquired immunity. Really?!? After more than two years of living with SARS-CoV-2 and and Delta and Omicron sweeping through Canada, one is to believe that only 20% have immunity? Where is the evidence for this? A paper showing that a vast majority of Canadians might have some degree of naturally acquired or cross-reactive immunity against SARS-CoV-2 can be found here. I would expect that even more unvaccinated Canadians would now have natural immunity. Unfortunately, the Canadian taskforce for gathering data about immunity among our population was disbanded so we missed the boat on tracking this incredibly important parameter that would have a major influence on any epidemiological model. So, what is the source of data used by Fisman, et al. to come up with their proposition of 20% immunity with a ‘plausible’ range of 10-50%? The source is stated as “assumption”! Isn’t that interesting. This is notable because all mathematical models are only as good as the assumptions that are plugged into them. The old adage is ‘garbage in, garbage out’. I will show you below how this single unjustified assumption biased the conclusions of the paper.
- Fatal flaw: “We treated immunity after vaccination as an all-or-none phenomenon, with a fraction of vaccinated people (as defined by vaccine effectiveness) entering the model in the immune state and the remainder being left in the susceptible state. For example, a vaccine that is 80% efficacious would result in 80% of vaccinated people becoming immune, with the remaining 20% being susceptible to infection.” There are multiple major issues here. First, the COVID-19 ‘vaccines’ fail to confer immunity, which is protection against disease and transmission. It is common knowledge that ‘vaccinated’ people get infected and sick at least as much as, if not more than the ‘unvaccinated’. This is why a desperate emphasis has been placed on the debatable possibility that disease severity is merely dampened by ‘vaccination’. A such, a biologically incorrect assumption was made here.Also, the effectiveness of the vaccine that was plugged into the model started at 40% and went up from there. However, these high percentages represent relative risk reduction, not absolute risk reduction. Do you remember when we were told that Pfizer’s ‘vaccine’ was 95% effective? That did not mean that 95% of the population was protected against getting COVID-19 (or 80% as used in the example in the paper). That 95% reduction was a relative risk reduction. What many people were not told is that most of the people in Pfizer’s clinical trial never got COVID-19, which is the disease that can occur in some people who get infected with SARS-CoV-2. In fact, the absolute risk reduction at the population level in the study was a mere 0.84% as a result of ‘vaccination’. As such, it is completely inappropriate for Fisman, et al. to plug values for relative risk reduction into their population-level models and treat them as though they represent absolute risk reduction. Conflating relative versus absolute risk reduction was misleading. As expert epidemiologists the authors must surely know the difference between absolute versus relative risk reduction. This is evidence that what they disseminated was disinformation.
- Fatal flaw: “We did not model waning immunity”. I was flabbergasted by this assumption. COVID-19 ‘vaccine’-induced immunity is ridiculously short-lived. In contrast, naturally acquired immunity is much longer-lived. This differential effect would have had a major influence on the outcome of the mathematical model. This assumption by the authors ignores obvious scientific facts.
- Major issue: There was no discussion about assumptions with respect to the safety (or lack thereof) of the COVID-19 ‘vaccines’ that might offset the perceived value of ‘vaccination’. Regardless of their viewpoint, they should have discussed this and provided evidence for their stance.
- Major issue: “Boosting with mRNA vaccines appears to restore vaccine effectiveness at least temporarily against Omicron”. This is not an accurate assumption to make. The paper that was cited to justify this statement used data derived from a simplistic model in a petri dish. It provided evidence of the presence of SARS-CoV-2-neutralizing antibodies in the blood of people who received COVID-19 booster ‘vaccines’. However, this lacked a functional context. First, these antibodies were measured in blood, but the virus infects the airways, so the anatomical location that was studied is irrelevant. Second, it is possible that there were non-neutralizing antibodies that could actually enhance disease but no testing was performed to detect these; non-neutralizing antibodies were ignored. There are other issues, but the point is that there was no way of knowing whether the subset of antibodies measured in the cited study would translate into any degree of protection against ‘real world’ infection. This shows a bias by Fisman, et al. in pushing booster doses with the weakest of data to support it.
- A refreshing truth: “The simplicity of our model is… a weakness, because it does not precisely simulate a real-world pandemic process in all its complexity”. This is an understatement and is one of the reasons why the paper should be retracted.
- Major issue: The paper treats COVID-19 ‘vaccines’ as though these are the only way to reduce the harm of COVID-19 among Canadians. There was no discussion of alternative strategies such as prophylaxis or treatment using re-purposed drugs, the promotion of vitamin D sufficiency (a vital molecule for optimal functioning of the immune system) among a population in a northern climate that is plagued with seasonal vitamin D insufficiency, etc.
- Major issue: Fisman declared the following competing interests: “David Fisman has served on advisory boards related to influenza and SARS-CoV-2 vaccines for Seqirus, Pfizer, AstraZeneca and Sanofi-Pasteur Vaccines”! These seem like inappropriate conflicts of interest, especially in light of the major flaws in his paper. In combination, these are suggestive of an inappropriate bias in judgement.
- Minor issue: “He also served as a volunteer scientist on the Ontario COVID-19 Science Advisory Table”. My issue here is not related to a conflict of interest, but rather the shoddy epidemiological modeling in his current paper raises serious questions about all of the historical modeling done with the Ontario Science Table. Journalist Brian Lilley published an article in the Toronto Sun on March 16, 2022 about the poor track record of mathematical modeling by the Ontario Science Table (of which Fisman is a former key member of the modeling group). It included these quotes…“The self-appointed, self-important Science Table has been wrong with their modelling predictions more times, and in more ways, than I can count.”“The Science Table has somehow achieved a revered status in Ontario, a sort of secular sainthood bestowed upon their members and their work despite a spotty track record of predicting how the pandemic is going. They’re not an official government body; in fact, they have no official government role, but they are seen as and presented as the authoritative voice on COVID in the province.”“I’m just judging the Science Table by their track record and if you did that, you wouldn’t be listening to them either.”
- Major issue: The messaging in the paper by Fisman, et al. carries serious implications. It implies that the unvaccinated are selfishly causing harm to the ‘vaccinated’. If the conclusions of the paper were to stand, they would logically lead to things like policies for forced vaccinations or stricter segregation of the ‘unvaccinated’ or harsher penalties against them. It is scary to see scientists using disinformation to promote hatred and division amongst Canadians.
Proof that the Paper by Fisman, et al. Should be Retracted Immediately
For the final ‘nail in the coffin that is the paper by Fisman, et al., let’s make only one adjustment to their model. First, kudos to the authors for making their mathematical model available. Seeing the epidemiological models being used to inform COVID-19 policies has been a rarity over the past couple of years. Fisman did not make his model available in his previous paper (that was also published in the Canadian Medical Association Journal), nor for any models that he was involved with when serving on the Science Table. Unfortunately, the current disclosed model highlights exactly why nobody should ever trust any epidemiological model that has not been fully disclosed. I encourage you to download the model. You can find it as a Microsoft Excel file here.
Now select the first tab, entitled “Patch Model”. In the top left corner of this page you will see “Model Symbology and Parameters”…
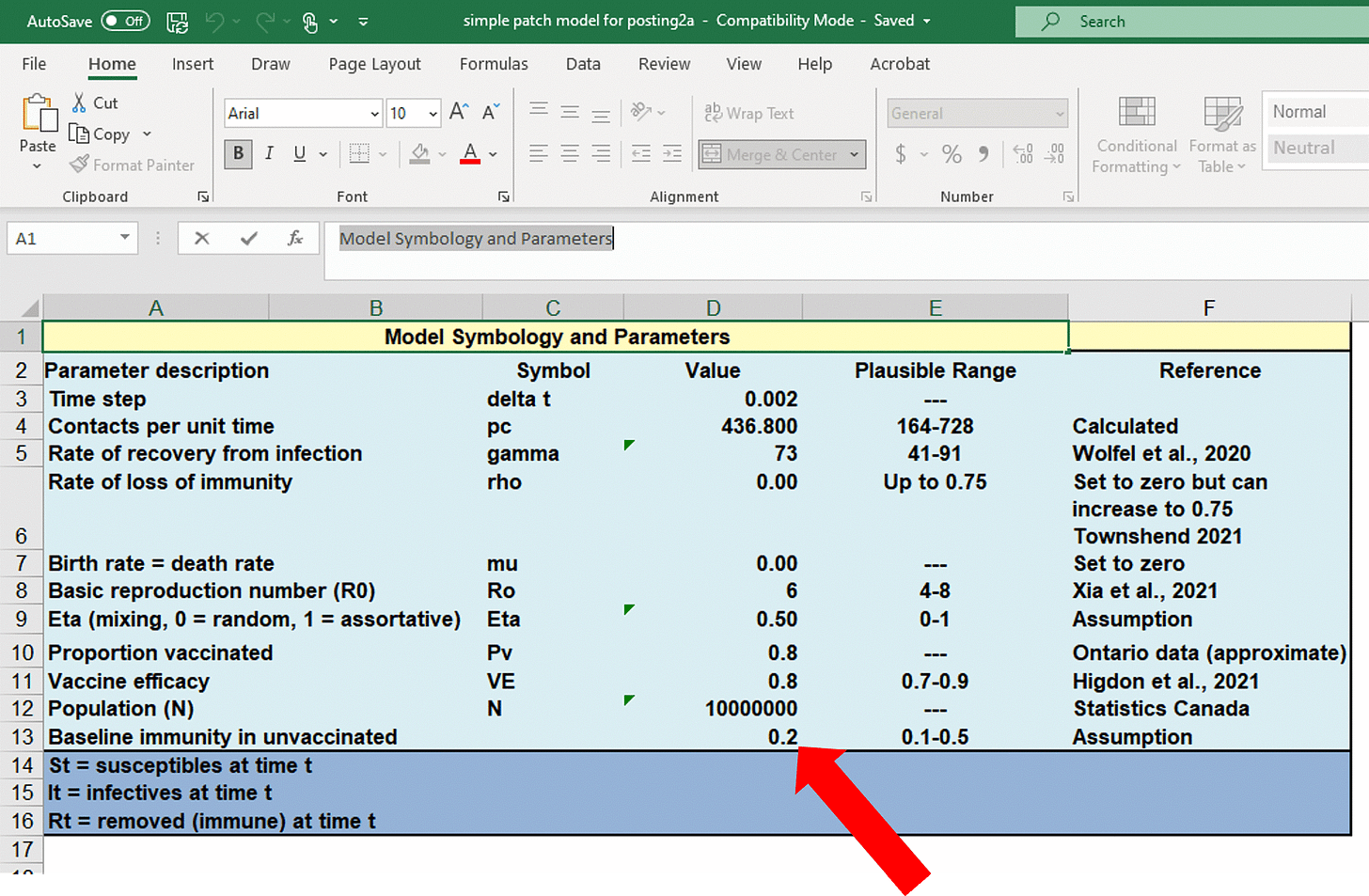
Note that the default value used for baseline immunity among the ‘unvaccinated’ was a mere 20%, which was based on pure speculation (se the ‘Reference’ column, cell F13). Remember, I cited a peer-reviewed published scientific paper suggesting that ~90% have immunity.
If you scroll to the right on the same Excel page, you will find the following data starting at cell AD19…
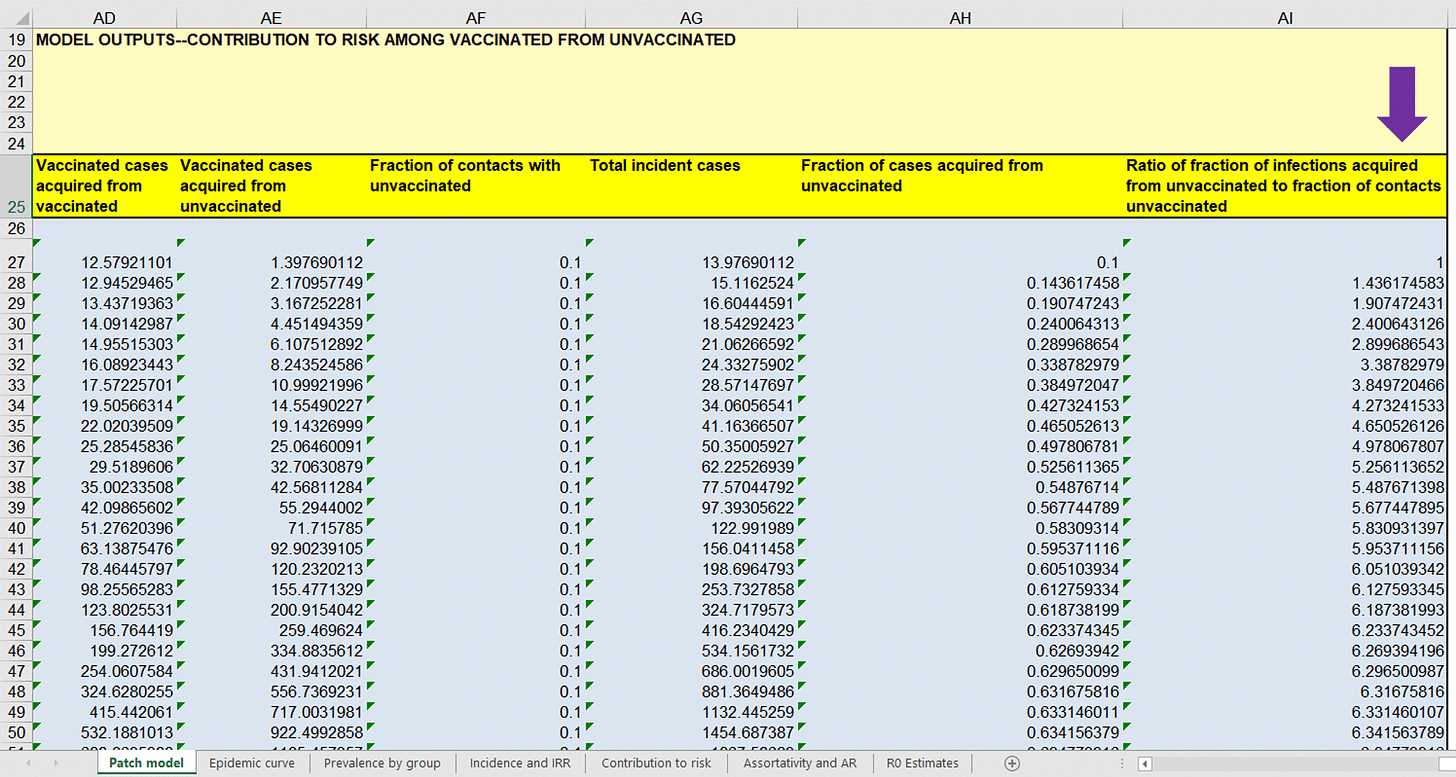
…below the purple arrow are numbers that provide a theoretical indication of the proportion of cases of COVID-19 that ‘vaccinated’ people got from the ‘unvaccinated’ after normalizing for their amount of contact people with the ‘unvaccinated’ group. A number larger than ‘1’ indicates that cases of COVID-19 among the ‘vaccinated’ came disproportionately from contact with the ‘unvaccinated’. The first row of data represents the starting point of a theoretical wave of COVID-19, hence the reason why column AI27 starts at ‘1’. As you move down the column, the time into the modeled wave of cases of COVID-19 increases. Note that the ratios rapidly rise well above ‘1’, suggesting a bias in transmission coming from the ‘unvaccinated’. This is the basis for Fisman, et al. promoting fear of the ‘unvaccinated’.
However, I have provided an excellent-quality peer-reviewed scientific article as a reference that suggests that immunity among ‘unvaccinated’ people in British Columbia was ~90%. To be conservative, let’s say it is only ~85% across Canada, despite this likely being an underestimate as most Canadians were exposed during the record-shattering waves caused by the Delta and Omicron variants sweeping through the country. So, let’s change this single parameter in the mathematical model to see what happens. I changed the proportion of the ‘unvaccinated’ with immunity from 20% to a justifiable 85%…
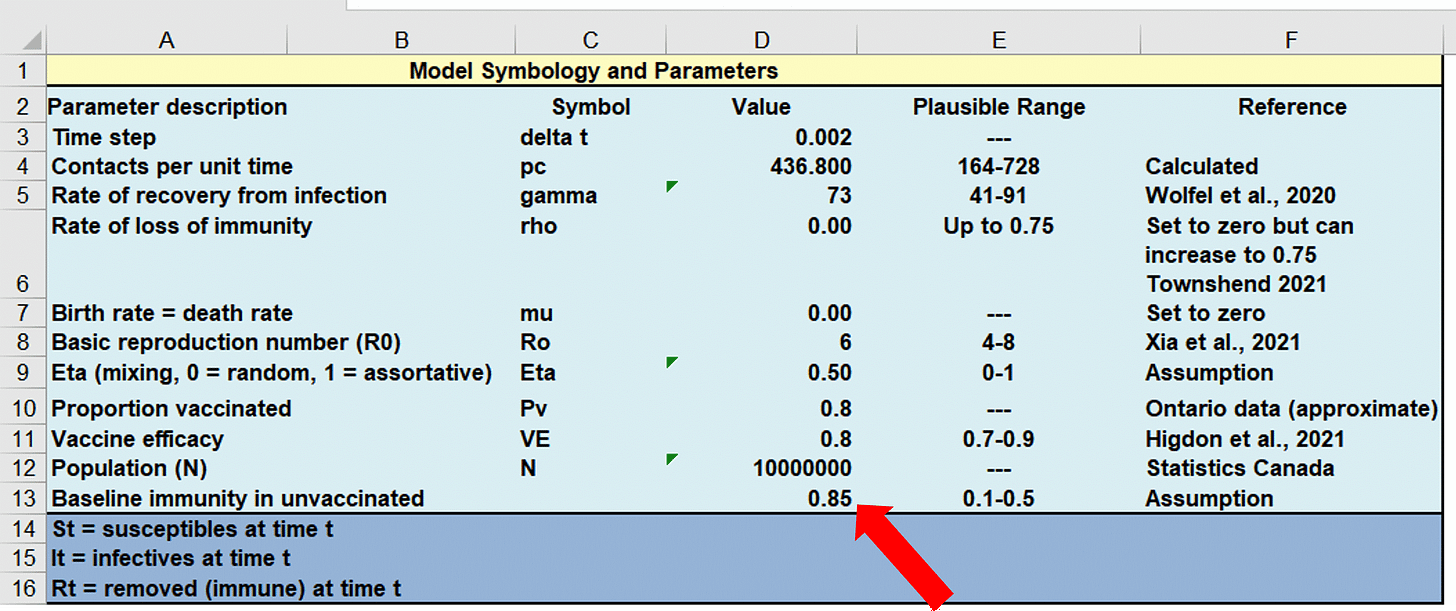
…now look at the effect this had on the data (look under the purple arrow)…
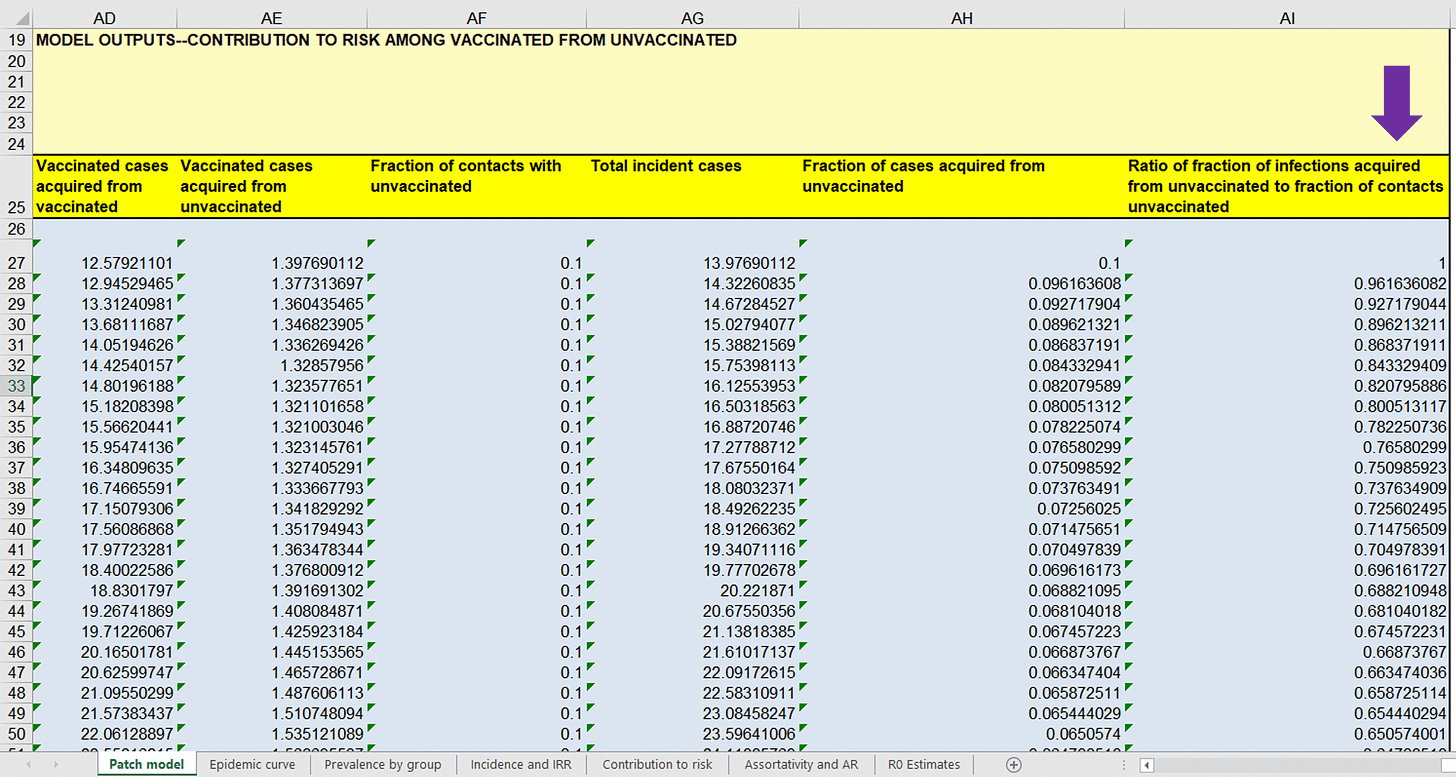
…remarkable, isn’t it! Correcting only this one assumption completely reverses the conclusions of the paper. All of a sudden every ratio drops below ‘1’, meaning that transmission is occurring disproportionately from ‘vaccinated’ people. Now the ‘unvaccinated’ are serving as a protective buffer for the ‘vaccinated’.
Now one must ask how skewed the conclusions of the paper would go in the opposite direction if the several other incorrect assumptions were to be corrected. As you can plainly see, this paper by Fisman, et al. is nothing short of preposterous. How could a paper be allowed to be published in what used to be a respected medical journal when its conclusions get completely reversed when only one of several reasonable corrections are made! The paper by Fisman, et al. is a classic demonstration of ‘garbage in, garbage out’. The authors are very intelligent, well-trained scientists who ply their trade well. They know what they are doing. As such, in my expert opinion, their paper represents disinformation (i.e., the deliberate dissemination of misinformation). I would be willing to stand in a court to justify my opinion. I honestly believe that the authors need to be investigated, as does the editor who allowed the paper to be published; and it would be great if the names of the reviewers who supported its publication could be made public. And the legacy media outlets that are promoting this ‘scientific’ garbage should be ashamed. They could use some immunology expertise and a return to investigative journalism to separate themselves from being labeled as propaganda artists.
Modeling Should Predict ‘Real World’ Data
Here are some ‘real world’ data that Fisman, et al. would be wise to consider in their modeling. This was copied from the website of the Ontario Ministry of health on April 26, 2022…
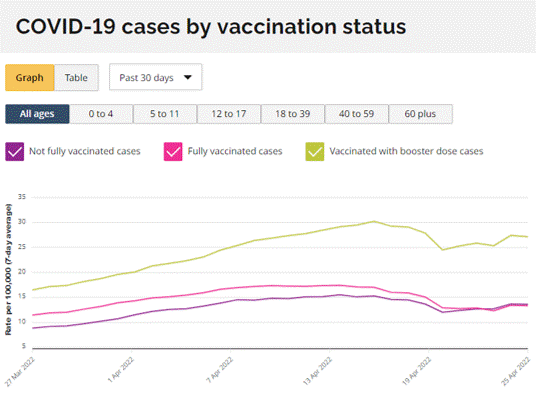
At the end of their paper, Fisman, et al. strongly promoted booster doses as a way to reduce ‘infections’. Yet the ‘real world’ data clearly show that the boosted sub-population is being diagnosed with disproportionately more cases than the ‘not fully vaccinated’ group, which includes the ‘unvaccinated’ and people who received a single dose. Why would someone want to take a booster and more than double their risk of getting diagnosed with COVID-19?!? Which is stronger evidence, the public health data shown above or contradictory messaging based on inappropriate massaging of a purely theoretical model? When mathematical models are misused they become no more utilitarian than toys.
A Direct Message to Fisman, et al.
Fisman and Tuite: Your paper, which can be flipped on its head by correcting just one of your multiple incorrect immunological assumptions, stigmatizes the ‘unvaccinated’ and could potentially be used to justify policies as draconian as forced inoculations. Why are you even promoting messaging based on theoretical models using assumptions that you are not qualified to opine on when concrete scientific evidence about transmission could be generated via biological sampling? You owe Canadians an apology for disseminating harmful disinformation. Should you wish to contest my immunological critiques, I would invite you to arrange a forum where we can have an objective third party moderate a respectful discussion about COVID-19 vaccinology in front of the Canadian public with equal representatives on both sides of the debate.
A Message to the Administration of the University of Toronto
You should launch an investigation into the academic conduct of Drs. Fisman and Tuite.
A Message to the College of Physicians and Surgeons of Canada
You should investigate Dr. Fisman and the harms that may be caused by his actions as a physician in publishing a misleading scientific paper in a medical journal.
A Message to the Canadian Medical Association Journal
Do the right thing and immediately retract the paper by Fisman, et al. There is a rumour that you may have sent this paper directly to Canadian physicians. If true, make it very clear to them that the paper represented disinformation disseminated by a physician who knows better. You should reconsider future recruitment of the services of the reviewers that promoted the publication of this paper and recommend that their academic institutions review their conduct. You should also review the conduct of your editorial board and peer review process.
A Message to the Legacy Media
Make the right choice and do everything that you can to blunt the profound harms caused by your rampant and widespread dissemination of misinformation. It is promoting hatred against critically thinking people who made highly informed and justified choices to avoid inoculations that are still in their initial clinical experimentation phase and for about which there is profound scientific debate. Protect the people that you have inappropriately placed at risk. You know where it will lead if you promote polarization of two groups of people and fuel feelings of anger and hatred in one of them against the other. Replace journalists with those who are willing to think critically and who will not support censorship of legitimate experts with ‘dissenting’ views. Nor should peer-reviewed scientific papers be treated like the gospel truth. The anonymous peer review process is fallible.
A Message to All Canadians
We are more alike than different. Do not fall into the trap set in the paper by Fisman, et al. to equate ‘likeness’ with ‘vaccination’ status. As an expert vaccinologist who has been closely following the accumulating science and, more importantly, as a fellow human being, I implore you to promote unity.
Correcting the Disinformation
Fisman had Tweeted, “Our paper supports the idea that the decision to remain unvaccinated confers risk not only on the unvaccinated individual but (disproportionate to contact rates) on vaccinated individuals too”.
If Fisman were to demonstrate objectivity as a scientist, he would update his Tweet to state something like the following: “After correcting just one of the several inappropriate immunological assumptions, our paper now supports the idea that the decision to get ‘vaccinated’ confers risk not only on the ‘vaccinated’ individual but (disproportionate to contact rates) on ‘unvaccinated’ individuals too; unlike our previous conclusion, this corrected model matches real-world data. Thank-you to those who chose to remained ‘unvaccinated’ since you are now selflessly serving as a buffer to the ‘vaccinated’. We are sorry to the field of public health modeling for disclosing how easy it is for the conclusions of our models to be manipulated by assumptions that we sometimes pull out of thin air. We also apologize to ‘unvaccinated’ people (most of whom have received legitimate vaccines throughout their lifetimes) for misleading media organizations around the world into promoting hatred against you. Now that our model has been shown to point to the ‘vaccinated’ as the main culprits of transmission of SARS-CoV-2, we implore you to not promote hatred against us like we have done to you.”
…don’t worry Dr. Fisman, I can tell you sincerely and from experience that the vast majority of ‘unvaccinated’ people will not return ill wishes in kind, even as more hypocritical messaging is unveiled. I for one want to see unity restored among Canadians. Hatred, segregation, and mislabeling of people have no place here. Much healing needs to happen and your current misdeed has been very counterproductive.
Overall Conclusions
No government should be allowed to implement policies based on results of epidemiological modeling prior to full disclosure of the data, the model used to generate predictions, and the conflicts of interests of the modelers. As demonstrated by Fisman, et al., public health models in the hands of the wrong people can result in devastatingly harmful and brutally misleading public messaging. This, in turn, could lead to the introduction of inappropriate health policies. And finally, multiple objective immunologists should always be consulted to advise on the immunological parameters being plugged into any health-related models.
In the name of good science, the correction of mis/dis-information, and in a desperate attempt to maintain some public faith in vaccinology,
Byram
To CMAJ and Mainstream Media Editors
Written by Ted Kuntz
The April 25, 2022 paper by Fisman et al undermines trust in our medical system, regulatory system, public health and now the Canadian Medical Association.
Fisman et al. (2022) Impact of population mixing between vaccinated and unvaccinated subpopulations on infectious disease dynamics: implications for SARS-CoV-2 transmission represents an abject failure of the obligation of the CMAJ’s editors/peer review process to scientific integrity.
The Fisman et al. paper is a thinly disguised effort to inflame the public into reacting against those who have consciously chosen not to partake in the experimental genetic technology fraudulently called a vaccine.
The CMA, CMAJ and our media need to be held accountable for this egregious violation of fundamental principles of good science and responsible journalism.
An immediate retract by the CMAJ is needed, as is an apology from all media that naively chose to endorse such obvious efforts to divide our Nation.
I fully expect the CMAJ and the media will share the following critical assessments of the Fisman study in their retraction and apologies.
Sincerely
Ted Kuntz
1. Dr Bridle: Fiction designed to promote hatred https://viralimmunologist.substack.com/p/fiction-disguised-as-science-to-promote
3. OCLA Statement on CMAJ Fisman et al. Article Claiming Disproportionate Infection Risk from Unvaccinated Population, and on Negligent Media Reporting https://ocla.ca/ocla-statement-on-cmaj-fisman-et-al/
4. Lawyer Knia Singh: Human rights and legal critique of the recently published article regarding the impact of viral transmission between the vaccinated and the unvaccinated. https://www.instagram.com/tv/CcyxL2RlSc4/?utm_source=ig_web_copy_link
5. Jestre: Impact of population mixing: The tractability problem https://jestre.substack.com/p/impact-of-population-mixing-the-tractability?s=r
6. David Chesnut: New Study Claiming Unvaccinated Spread COVID More Than Vaccinated – Pseudoscience, Propaganda, and Misleading Lies https://www.facebook.com/101852609908112/posts/5130922020334454/?sfnsn=mo
8. Press: Life Site News https://www.lifesitenews.com/blogs/canadian-doctor-compares-unvaccinated-to-murderous-drivers-citing-insane-study/